AI-powered medicine

August Hays-Ekeland’s research with the Neural Engineering Lab focuses on the part of the brain responsible for speech output. Photo by Jeff Newton
Editor's note: This story originally appeared in the summer 2024 issue of ASU Thrive magazine.
The human body is dripping in data, from the steady beat of the heart to electrical impulses shooting through the brain, to the myriad ways we walk and talk.
Tapping into that information to treat patients is one of the great challenges faced by doctors all over the world — but it’s being made easier thanks to advances in artificial intelligence.
ASU researchers are at the forefront of this new wave of medical research. Here are just some of the ways they are exploring how AI and machine learning can be used to speed up diagnosis, unlock new treatments and access new data sources that could shed light on the mysteries inside us all.
Assessing treatments for neurological conditions
Speech demands precise choreography — tongue, lips and jaw moving in harmony. But in neurological conditions like Alzheimer’s, Parkinson’s and ALS, those movements break down.
Breaking new ground
Learn more about AI at ASU at ai.asu.edu.
Sufferers may experience slurred and slowed speech, difficulties in recalling words, and instances of incoherent speech, says Visar Berisha, associate dean and professor in ASU’s Ira A. Fulton Schools of Engineering and professor in the College of Health Solutions. Berisha and Julie Liss are developing AI models that analyze both the content of someone’s words and the way they sound to “reverse engineer” what’s happening.
“The speech signal is very rich and has a lot of information about the state of a person and their health and emotions,” says Liss, an associate dean and professor in the College of Health Solutions.
In 2015, Liss and Berisha co-founded a spinout company to bring the insights to patient care. While some startups are trying to use speech to diagnose conditions like Alzheimer’s, Liss and Berisha are taking a more conservative approach with potential for immediate impact.
Across many neurodegenerative conditions, there is a high failure rate in clinical trials. This is because the tools used to evaluate whether patients are improving from treatments are coarse and subjective. To solve this, Berisha and Liss use their AI model to evaluate whether patients are responding to treatment in clinical trials, with success already demonstrated in prospective trials in ALS.
These and other successes have led to breakthrough device designation status from the Food and Drug Administration, device registration with the FDA and global adoption by pharmaceutical companies and health care providers to track patient health.
“Speech analytics provide objective, interpretable, clinically meaningful measures that allow for clinical decision-making,” says Berisha. The hope is that AI will be able to pick up subtle changes in speech that a clinician might have missed.
Standardizing brain scans
Inside the brain, Alzheimer’s is characterized by the growth of amyloid plaques, clumps of abnormal proteins that form in the gaps between neurons. New treatments such as lecanemab target these plaques. To track how these drugs are working, subjects in clinical trials are injected with a radioactive tracer that sticks to the amyloid so that it shows up on a PET scanner.
There are five different FDA-approved tracers, all with different properties, and different clinics use different tracers, making it hard for researchers to compare data. AI can help.
Teresa Wu, a professor in ASU’s School of Computing and Augmented Intelligence and health solutions ambassador in the College of Health Solutions, uses AI to harmonize PET scans taken with different types of tracers.
Wu’s model generates what a brain scan taken using one type of tracer would look like if it had been taken with a different one, enabling easier comparison and research.
“If you want to make use of imaging data to support medical decisions, there is a hurdle to overcome,” she says. “You cannot just take the data from different sources and dump it in your deep-learning model. You have to make sure it’s clinically relevant and usable.”
Doing that opens a wealth of new opportunities for using AI to improve diagnosis.
Another project uses AI and deep learning to predict a person’s biological age from MRI brain scans.
“With healthy subjects you expect their biological age and their true age to be similar,” Wu says.
If it’s not, that could be an early sign of neurodegenerative disease.
Plugging into the brain
Locked-in syndrome, caused by damage to the brainstem, stops signals from the brain from reaching the rest of the body. The person can see and hear, but they’re robbed of their ability to move, speak and communicate through anything but eye movements and blinking.
Bradley Greger, an associate professor in ASU’s School of Biological and Health Systems Engineering, is using artificial intelligence and machine learning to translate what’s happening in the brain in the hope of helping people with locked-in syndrome communicate more fluidly.
“It’s about tapping into those areas of the brain that process language and speech, recording the neural activity, and then using machine learning algorithms to figure out how that neural signal maps to the word they’re trying to speak,” he explains.
The research piggybacks on patients who are already having surgery to place electrodes in their brain to treat conditions such as epilepsy. Greger says this is the only way to get the detailed level of information needed — noninvasive brain scanning techniques like EEG and fMRI aren’t precise enough.
Machine learning really helps with processing the huge amount of data that’s generated.
Bradley GregerAssociate professor in ASU’s School of Biological and Health Systems Engineering
That information then can be used to train a model to predict what a person is trying to say.
Greger also notes that the brain is active while listening to speech — even in patients who are locked in.
“Ultimately, we’ll be able to understand not only what the patient is saying, we’ll also be able to confirm cognitive understanding when listening to speech,” he says.
Greger hopes to use this research for other applications: enabling paralyzed people to control a robotic limb, for instance, or blind people to see with the aid of a camera connected to the retina, optic nerve or visual processing areas of the brain.
“For somebody who’s blind or paralyzed, it can be really life-changing for millions of people,” he says.
“Machine learning really helps with processing the huge amount of data that’s generated — terabytes of data,” Greger continues. “And then you have to map that onto the person’s behavior and what they’re saying.”
Training therapists
How do you train a therapist? You start by hiring actors. Right now, practice patients played by budding TV and movie stars are one of the best ways to re-create scenarios a therapist might face in sessions.
But there are problems with that approach. First, how do you get the training to people in remote areas who don’t have a supply of actors? How do you make sure the actors provide the same experience?
Psychologist Thomas Parsons, the Grace Center Professor of Innovation in Clinical Education, Simulation Science and Immersive Technology, is using AI to create virtual standardized patients that he hopes will solve some of 9these problems.
Parsons, an Air Force veteran, is particularly interested in helping clinicians better treat ex-military patients with blast injuries, combat stress symptoms and insomnia.
He’s the lead investigator on a more than $5.2 million project supported by the Department of Defense Congressionally Directed Medical Research ProgramsThe work was supported by the Assistant Secretary of Defense for Health Affairs endorsed by the Department of Defense, in the amount of $5,072,397.00, through the Congressionally Directed Medical Research Programs Peer Reviewed Medical Research Program under Award No. HT9425-23-1-1080. Interpretations, conclusions and recommendations are those of the author and are not necessarily endorsed by the Assistant Secretary of Defense for Health Affairs or the Department of Defense. that trains an AI virtual patient using transcripts and video recordings of sessions between real patients and doctors. That will be combined with digitized recordings of actors to create realistic virtual patients who can say what a real patient would say.
Eventually, Parsons says, the system will be able to assess the clinicians’ performance and adapt.
“There are specific things that you’re supposed to ask in a structured clinical interview, and we can look at how the human is deviating from that,” he says. “And then there’s six sessions. So, over six sessions you want to see this virtual patient get better.”
Mapping cancer cells
In the early days of biology, scientists would dissect and examine, peer through microscopes and draw sketches of what they saw.
“It was easy for them to keep in their heads how things work,” says Christopher Plaisier, an assistant professor in ASU’s School of Biological and Health Systems Engineering in the Fulton Schools of Engineering.
Today that’s impossible. We now know there are 25,000 genes in the human genome, and 9,000 of them are expressed in any given cell. The only way to understand the relationships between those genes and how they interact with diseases like cancer is with the help of AI.
Plaisier is trying to build what he calls “intelligible systems.” These AI models will classify different types of cells and help scientists understand the underlying biology better.
Techniques like unsupervised learning can find similarities between different cell types and map out the complex genetic pathways that govern their growth. That can unlock exciting opportunities for medicine.
In one strand of work, Plaisier used AI to analyze tumor cells from malignant pleural mesothelioma, a type of lung cancer. By tapping into databases of existing drugs, his team found 15 FDA-approved drugs that could potentially be repurposed to treat this condition.
Another project looks at quiescent cancer cells, which are in a dormant, nonreplicating state but could start growing again at any time. Plaisier is using neural network-based classifiers to map the cell cycle of these cells, in the hope of finding ways to knock tumors into quiescence so they stop growing, or out of quiescence so they’re more susceptible to chemotherapy.
AI will help researchers make medical discoveries that weren’t possible before, Plaiser says.
“We’re trying to understand how biological systems work, and that’s going to lead us to a place where we can actually start to make decisions intelligently,” Plaisier says. “That’s where machine learning methods can really help us dig in.”
Preventing falls in seniors
For seniors, falls pose a risk that can derail their ability to live on their own. To try to help, ASU Professor Thurmon Lockhart in the School of Biological and Health Systems Engineering has added AI to his biomechanics research.
“Traditional fall-risk assessments for seniors don’t always target specific types of risk, like muscle weakness or gait stability,” Lockhart says.
He’s developed a wearable device that goes across a patient’s sternum. It measures body posture and arm and leg movements in real time to monitor fall risk at home. When the risk is deemed to be high, a smartphone app called the Lockhart Monitor can alert the user or a caregiver before someone actually falls.
“This integration allows for real-time customization of individual patient-care pathways, all tracked through a centralized data system and reported to a clinical team to enhance the ability to improve patient outcomes and target high-risk patients to reduce avoidable injuries,” Lockhart says.
We’re trying to understand how biological systems work, and that’s going to lead us to a place where we can actually start to make decisions intelligently.
Christopher PlaisierAssistant professor in ASU’s School of Biological and Health Systems Engineering
Revolutionizing medicine
These projects represent only some of ASU’s work using AI to make medical breakthroughs.
As sensors get cheaper to make and artificial intelligence tools become easier to use, more medical fields will be able to unlock the power of big data. AI is already helping people receive better care. In the future, it could unlock a golden era of medicine for all.
Story by Amit Katwala. An editor and writer at WIRED, Amit Katwala works on features, science and culture. His latest book is “Tremors in the Blood: Murder, Obsession and the Birth of the Lie Detector.”
More Science and technology
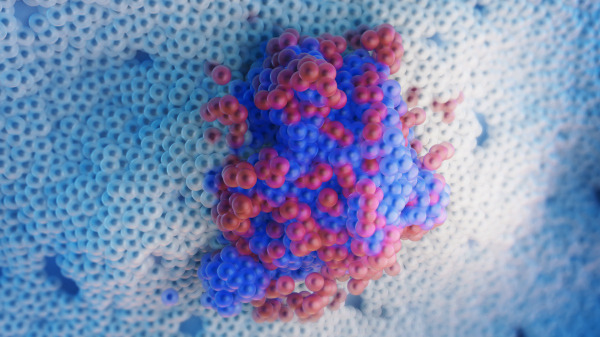
From food crops to cancer clinics: Lessons in extermination resistance
Just as crop-devouring insects evolve to resist pesticides, cancer cells can increase their lethality by developing resistance to treatment. In fact, most deaths from cancer are caused by the…

ASU professor wins NIH Director’s New Innovator Award for research linking gene function to brain structure
Life experiences alter us in many ways, including how we act and our mental and physical health. What we go through can even change how our genes work, how the instructions coded into our DNA are…

ASU postdoctoral researcher leads initiative to support graduate student mental health
Olivia Davis had firsthand experience with anxiety and OCD before she entered grad school. Then, during the pandemic and as a result of the growing pressures of the graduate school environment, she…