Sparking an evolution in robotics

Arizona State University Assistant Professor Zhe Xu (far right) is pictured on ASU’s Tempe campus with students working on a testbed for a Stretch 2 robot, which can operate autonomously, and a quadrotor, a robotic helicopter often used for mapping and rescue operations. A National Science Foundation CAREER Award will support further research led by Xu aimed at advancing cyber-physical systems. The project will involve the application of reinforcement learning, causal inference and control theory to enhance the learning abilities and performance of robotic technologies. Photo courtesy of Zhe Xu/ASU
Thinking about swarms of robots might conjure up images from old sci-fi movies in which Earth is invaded by armies of mechanical androids from another world that are programmed and weaponized to subjugate humans.
For Zhe Xu, what comes to mind instead is the progress he is striving to make in robotic systems that would help enable life-enhancing technological breakthroughs.
Xu is an assistant professor of mechanical and aerospace engineering in the School for Engineering of Matter, Transport and Energy, part of the Ira A. Fulton Schools of Engineering at Arizona State University. His studies have spanned mechanical, electrical, computer and systems engineering, as well as automation engineering.
His endeavors in robotics today focus on cyber-physical systems, which integrate computation, sensing, networking and control mechanisms into objects, machines and infrastructure. Cyber-physical systems also enable technologies and infrastructure systems to connect to each other and to the internet to make them more responsive, reliable, secure and efficient.
The National Science Foundation (NSF) foresees advances in these systems resulting in major leaps in the evolution of “smart” devices, transportation, robotic systems, power grids, smart cities and much more.
Expanding learning capabilities of vital technologies
Xu’s work has earned him an opportunity to aid in the pursuit of realizing such elevated aspirations.
He is the recent recipient of a 2024 NSF Faculty Early Career Development Program (CAREER) Award for a project titled "Temporal Causal Reinforcement Learning and Control for Autonomous and Swarm Cyber-Physical Systems." The award will provide about $550,000 over five years for Xu’s research.
Reinforcement learning is a kind of machine learning that enables systems driven by artificial intelligence, or AI, technology to educate themselves by trial and error based on feedback from their actions.
“Broadening these learning capabilities would be transformative for a wide range of cyber-physical system applications,” Xu says. “It would enable more efficient and effective decision-making processes by autonomous and swarm cyber-physical systems, such as self-driving cars, drones, industrial robots, swarm robots and power systems.”
Xu sees possibilities for further research to discover ways to enable such systems to not only maintain but also improve the safety and reliability of autonomous vehicles and other robotic systems, thus reducing the risk of malfunctions and accidents.
Another goal is to develop larger-scale applications of these systems that are capable of effectively handling more technologically complex tasks.
“That will make it easier for nonexperts to train and deploy robots and autonomous systems,” Xu says. “This could empower both large industries and small businesses to leverage these kinds of advanced AI-based cyber-physical system technologies, which ideally would lead to more investment, a more competitive marketplace and more innovation.”
Paths to advanced intelligence in robotics
Yi “Max” Ren is an associate professor of aerospace and mechanical engineering in the School for Engineering of Matter, Transport and Energy, and director of ASU’s Design Informatics Laboratory. He works with Xu on imperfect-information games, which challenge defenders to identify and cope with deceptive behaviors by opponents with hidden intents.
The uniqueness of Xu’s research is illustrated by an example he gave in one of his recent research papers, Ren says.
Ren explains it this way: Let’s say a robot is tasked to pick up either a cup of coffee from room A or a soda from room B and bring it to the person in room C. The robot also knows that if it goes into room B, it will get locked in. Therefore, it deduces that the only option is to pick up the coffee.
Conventional reinforcement learning focuses more on computing a solution rather than on reasoning about the solution space. In this case, reinforcement learning will explore both options and gradually reduce the chance of picking up the soda because every time the robot does so, it fails in its mission.
“Xu is incorporating reasoning into computation. If there is no way to go from room B to C, we should not explore that option in the first place,” Ren says.
“Combining neural and causal computation is the path toward real intelligence. An example is AlphaGeometry, which solves International Mathematical Olympiad geometry problems at a very high level. What Xu is doing is along the same direction in the context of robotics.”
Fulfilling smart systems’ promising potential
As his work moves forward, Xu intends to bring several engineering doctoral students onto his lab team in coming years to gain research experience in cyber-physical systems and other fast-emerging tech fields related to these systems.
He also plans to promote expansion of efforts to teach Fulton Schools students about AI-assisted adaptive and interactive learning. It’s important to provide them more knowledge in areas that he expects to become increasingly applicable to a variety of engineering pursuits, he says.
Xu foresees advances in cyber-physical systems enlarging the horizons of human interactions with technologies and engineered systems in ways that will impact almost every aspect of society.
Particularly when applied to robotics, improved cyber-physical systems will enable swarms of robots to be designed and programmed to perform a wide range of operations more effectively than current systems, he says.
He also says research is already demonstrating the potential for these systems to have applications in advancing manufacturing, medicine and health care, environmental sustainability, energy production and distribution, agriculture, aeronautics, building design and bionics to help restore people’s physical abilities.
Xu also expects cyber-physical systems to become a valuable tool in education.
Better robotics through temporal causal reasoning
The improvements researchers anticipate being made to these systems will boost the performance of robot swarms in search-and-rescue missions, surveillance systems and critical security networks, Xu says.
He emphasizes that new generations of cyber-physical systems will not only improve the mechanistic capabilities of these systems but also enable them to learn how to improve their effectiveness based on what they experience in undertaking their assigned missions.
“The systems’ behavior will be reinforced by good outcomes while they are also learning to avoid behavior that led to bad outcomes, and incorporating temporal causal knowledge can potentially make such learning more efficient,” Xu says.
That type of complex functionality is at the root of the temporal causal reinforcement learning and control abilities that Xu and his colleagues are working to take to the next level in larger and more complex robotic systems.
“What we want are robots that act and react logically,” he says, “robots that are capable of reasoning and prepared to make accurate and nuanced decisions in complex, dynamic and changing environments.”
More Science and technology
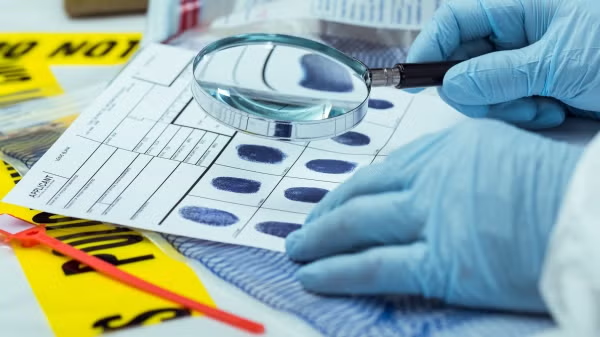
Learn about the secrets of forensic science straight from the experts
Over the next week, true crime enthusiasts will have a rare opportunity to discover the secrets of forensic science as experts share techniques for tracking down criminals.Behind Crime Scene…

New cancer treatment disrupts tumor growth
A new discovery may bring science closer to stopping cancer in its tracks.Researchers with the Biodesign Center for Applied Structural Discovery at Arizona State University and the team of Professor…

Phoenix-based startup taps ASU alum to develop digital coach to help with menopause
It started with secret conversations behind the scenes at technology conferences.But Susan Sly and her colleagues weren’t discussing the latest operating systems or the newest releases of computer…