New statistical model improves the predictive power of standardized test scores
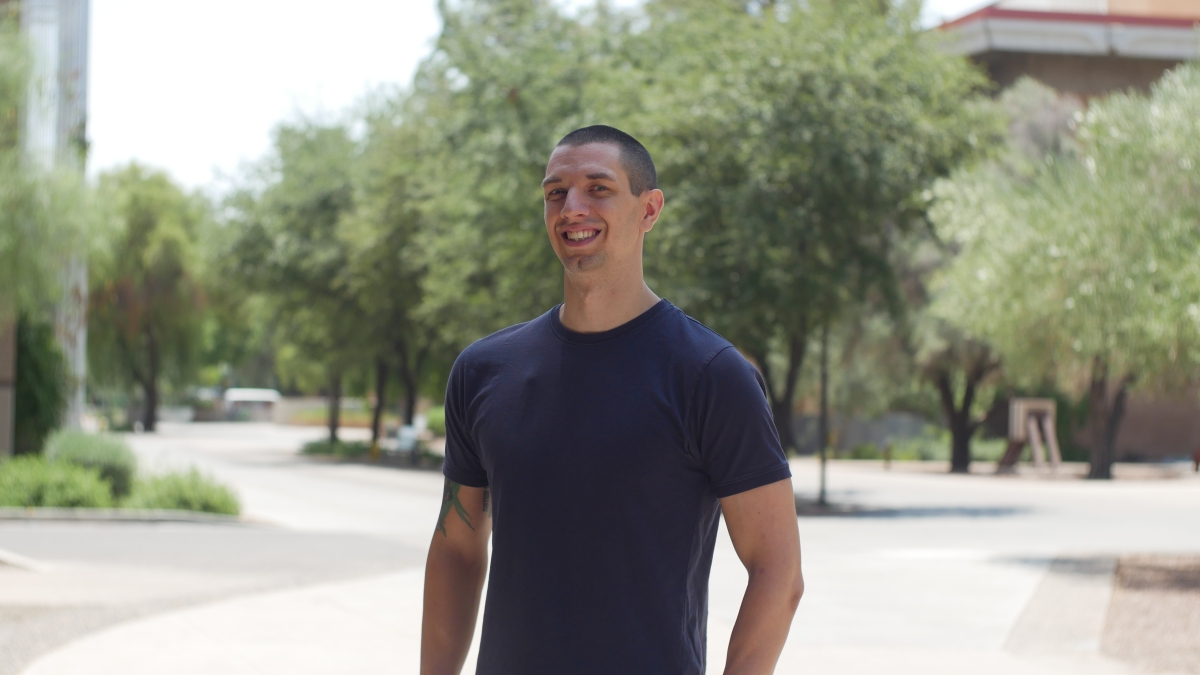
Daniel McNeish, assistant professor of psychology at ASU and first author on the paper. Photo by Robert Ewing
College admissions ask a lot: a standout essay, a high grade point average and stellar standardized test scores.
And the ongoing college admission scandal underscores how influential standardized test scores have become. A test administrator is now cooperating with the investigation into other parents who paid to have their children’s test scores fixed.
College admissions decisions use standardized test scores as a predictor of how well an applicant will do in college. But what if there were a better way to predict learning — one that did not rely on a single, high-stakes test?
Researchers from Arizona State University and the University of Denver have devised a way to predict academic performance that is three times more predictive than a single standardized assessment. The research team developed and validated a statistical model that uses readily available test scores to predict future academic performance. The study was published in Multivariate Behavioral Research on Nov. 21.
“Everyone is affected by testing at some point — tests are used to make high-stakes decisions about admissions to schools and sometimes even job placement — and the model we developed captures what is going on in the data and predicts future performance better than existing methods,” said Daniel McNeish, assistant professor of psychology at ASU and first author on the paper.
Current ability does not always predict future learning
The stated purpose of many standardized tests is a one-time assessment, not to inform long-term performance. These tests are sometimes used to predict the future performance of anyone who takes the test, but few tests actually do this well, said Denis Dumas, an assistant professor at the University of Denver and second author on the paper. The idea that a single test can fail to adequately measure a student’s future learning potential is not a new one: The sociologist, historian and civil rights activist W.E.B. Du Bois raised it almost a century ago.
“Test scores from a single time point give a good snapshot of what someone knows at the time of testing, but they often are incapable of providing information about the potential to learn,” Dumas added. “Test scores are frequently used to indicate how much a person might benefit from future education, like attending college, but this concept is completely different from how much the test taker knows right now.”
To develop the model, the research team took inspiration from the work of an Israeli psychologist named Reuven Feuerstein who tested children survivors of the Holocaust for school and grade-level placement. Grade-level assignments based on one test score were often too low, so Feuerstein developed a testing system called dynamic assessment that used several test scores collected over time to measure children’s capacity to learn, instead of their current level of knowledge. Dynamic assessment is labor-intensive and is difficult to implement on a large scale. The research team solved that problem by leveraging advances in mathematical models and computing power to create a new method, which they call a dynamic measurement model.
Connecting the dots
The dynamic measurement model uses a series of test scores to predict future learning capacity. The model fits a curve through the test scores over time, which usually looks like a sideways letter “J” and is often called a “learning curve.” The points on the learning curve represent the amount of current knowledge, and the maximum or ceiling of the curve is the learning potential. Using standardized test scores from kindergarten through eighth grade, the team recently showed the dynamic measurement model could fit the learning curve and predict learning potential.
The research team wanted to know how far out the model could predict learning potential and thus how accurate it actually was. They used three datasets that originated from the Institute of Human Development at the University of California, Berkeley. The datasets include test scores from participants starting when they were 3 years old in the 1920s and 1930s. The participants were studied for decades, until they were in their 50s, 60s, and 70s.
Because most standardized testing happens in school, the research team used the dynamic measurement model to fit the test scores from when the UC Berkeley participants were age 20 and younger. The team predicted the future learning potential of each participant by having the model finish the curve. Then, they compared the actual test scores at ages 50 to 70 years to what the model predicted.
“The dynamic measurement model captured three times the variance as other methods, including single time-point test scores. In other words, our model predicted the later scores — an individual’s realized learning potential — three times better,” McNeish said. “Students are tested so frequently now to gauge their progress, but having multiple scores per student can serve a purpose beyond gauging progress. They can be combined into a single learning potential score to improve predictions of where people’s skills and abilities are predicted to end up in the future if they maintain the same trajectory.”
Harnessing the potential of standardized testing
Using dynamic measurement modeling to predict the future learning potential of students does not require changes in policy or new tests. The test scores needed for the model already exist and are available because of the passage of the No Child Left Behind Act and Every Student Succeeds Act.
“Dynamic measurement modeling does not require a specialized computer to run and does not take much longer than standard statistical models used in this area,” McNeish said. “Logistically, all the pieces are there to implement it tomorrow.”
The research team is currently working on developing software to disseminate the dynamic measurement model.
ASU’s Kevin Grimm, professor of psychology, also contributed to this study.
More Science and technology
Extreme HGTV: Students to learn how to design habitats for living, working in space
Architecture students at Arizona State University already learn how to design spaces for many kinds of environments, and now they…
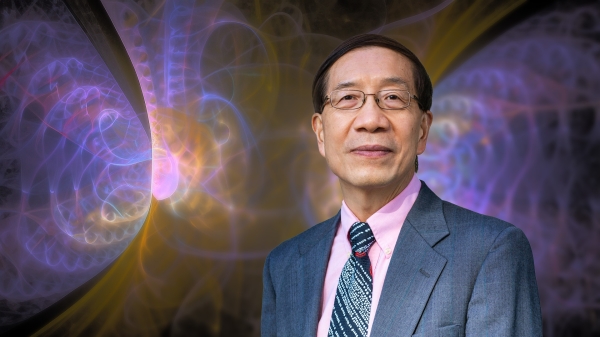
Human brains teach AI new skills
Artificial intelligence, or AI, is rapidly advancing, but it hasn’t yet outpaced human intelligence. Our brains’ capacity for…

Doctoral students cruise into roles as computer engineering innovators
Raha Moraffah is grateful for her experiences as a doctoral student in the School of Computing and Augmented Intelligence, part…