A leap of progress for energy-efficient intelligent computing
ASU engineer part of team exploring solutions to memory and energy challenges
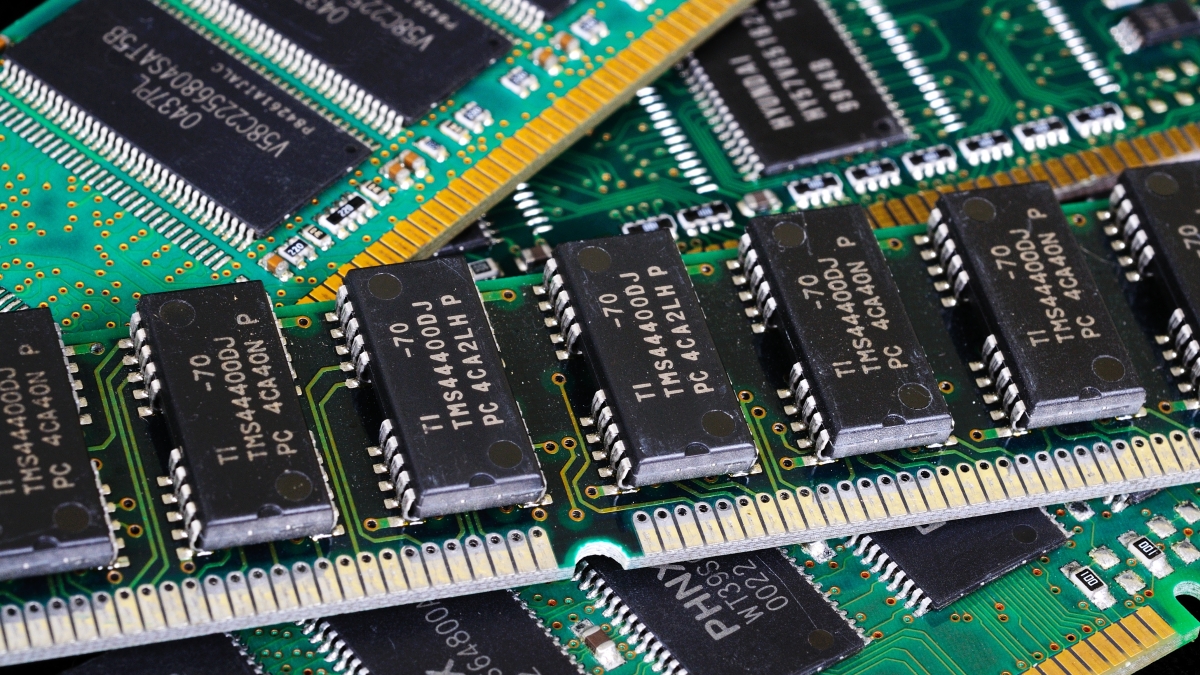
An assortment of random-access memory modules. Photo courtesy of Shutterstock
According to Andrew Ng, a pioneer in machine learning and co-founder of Google Brain, artificial intelligence will have a transformational impact on the world similar to the electricity revolution. From self-driving vehicles to smart biomedical devices, nearly all future technologies will incorporate algorithms that enable machines to think and act more like humans.
These powerful deep-learning algorithms require an immense amount of energy to manipulate, store and transport the data that will make artificial intelligence work. But current computing systems are not designed to support such large network models. Thus, innovative solutions are critical to overcoming performance-energy and hardware challenges, especially for memory.
The need for sustainable computing platforms has motivated Jae-sun Seo and Shimeng Yu, faculty members at Arizona State University and Georgia Tech, respectively, to explore emerging memory technologies that will enable parallel neural computing for artificial intelligence.
Neural computing for artificial intelligence will have profound impacts on the future, including in autonomous transportation, finance, surveillance and personalized health monitoring.
More sustainable computing platforms will also help bring artificial intelligence down to power- and area-constrained mobile and wearable devices, without employing a number of central and graphics processing units.
Seo and Yu’s collaboration began in ASU’s Ira A. Fulton Schools of Engineering, where Yu was a faculty member before joining Georgia Tech. Jeehwan Kim with the Massachusetts Institute of Technology and Saibal Mukhopadhyay with Georgia Tech round out their research team.
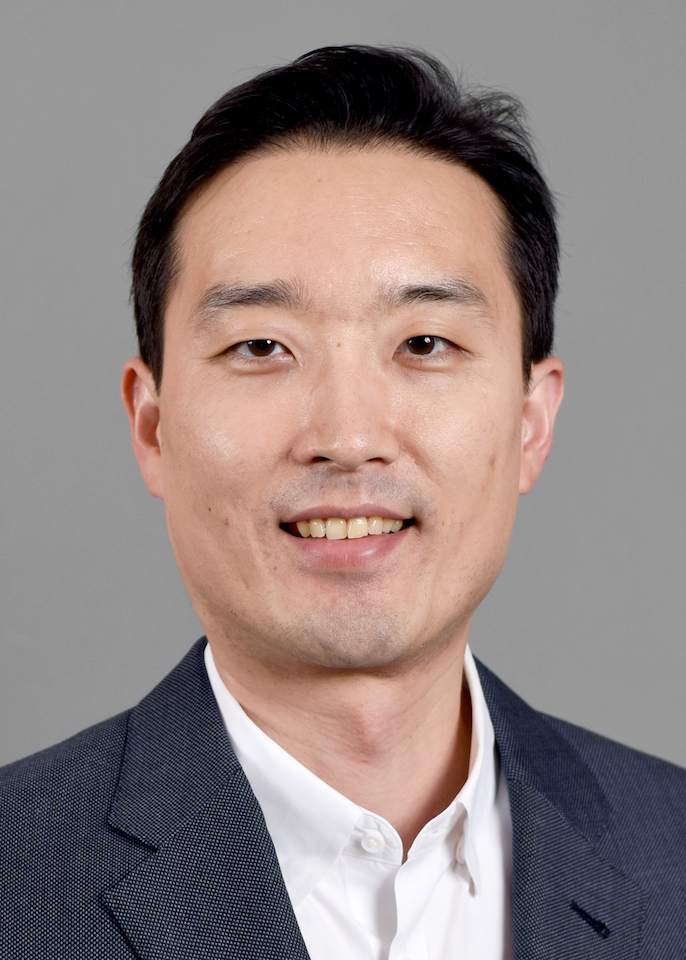
Jae-sun Seo
With ASU as the lead institution, the team received a three-year, $1.8 million grant from the National Science Foundation and the Semiconductor Research Corporation as part of the program called Energy-Efficient Computing: from Devices to Architectures, or E2CDA. The program supports revolutionary approaches to minimize the energy impacts of processing, storing and moving data.
“Overall, this project proposes a major shift in the current design of resistive random-access memories, also known as RRAMs, to achieve real-time processing power for artificial intelligence,” said Seo, an assistant professor in the School of Electrical, Computer and Energy Engineering, one of the six Fulton Schools.
The team wants to explore RRAM as an artificial intelligence computing platform — a departure from the way these applications are currently powered.
To accelerate deep-learning algorithms, many application-specific integrated circuit designs are being developed in CMOS, or complementary metal-oxide semiconductor, technology. CMOS is the leading semiconductor technology and is used in most of today’s computer microchips.
However, it has been shown that on-chip memory such as static random-access memory, or SRAM, creates a bottleneck in bringing artificial intelligence technology to small-form-factor and energy-constrained portable hardware systems based on two important aspects: area and energy.
For custom application-specific integrated circuit chips — which are used to power many artificial intelligence applications — cost limits the silicon space where information can be stored and restricts the amount of on-chip memory that can be integrated.
Considering that state-of-the-art neural networks, such as ResNet, require storage of millions of weights, the amount of on-chip SRAM is typically not sufficient and requires an off-chip main memory. Communicating data to and from the off-chip memory consumes substantial energy and delay.
SRAM is also volatile — and requires power to store its data. As such, it will consume static power and energy even when there is no activity.
As an alternative to on-chip weight storage technology with a higher density that is 10 times smaller than SRAM, emerging non-volatile memory devices have been proposed as a solution for long-term storage. Since the density is much higher, more weights can be stored on-chip, eliminating or substantially reducing high-energy dynamic memory communication.
Because non-volatile memory devices do not require a power supply to maintain the storage, static energy is also dramatically reduced. RRAM is a special subset of non-volatile memory devices that can store multiple levels of resistance states and could naturally emulate a synaptic device in neural networks, but it still has limitations for practical large-scale artificial intelligence computing tasks.
“We’ll perform innovative and interdisciplinary research to address the limitations in today’s RRAM-based neural computing,” said Seo. “Our work will make a leap of progress toward energy-efficient intelligent computing.”
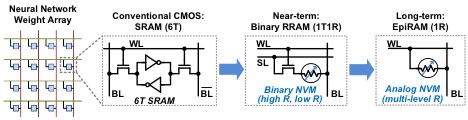
State-of-the-art neural networks require storage of millions of weights, while conventional SRAM storage is costly and consumes static power. Jae-sun Seo and Shimeng Yu’s E2CDA project investigates binary RRAM devices in the near term and analog RRAM devices in the long term as the storage and computing platform for artificial intelligence. Diagram courtesy of Jae-sun Seo
Seo and Yu’s research will address limitations in RRAMs by investigating novel technologies from device to architecture. Using a staged approach, in the near term, with today’s binary resistive devices (high and low resistance), the researchers will first develop new RRAM array designs that allow effective representation and parallel in-memory computation with positive and negative weights.
Over the longer term, the team will investigate a novel epitaxial resistive device, or EpiRAM, to overcome the material limitations that have been holding back the use of RRAM arrays as an artificial intelligence computing platform. The preliminary results of EpiRAM have shown many idealistic properties, including suppressed variability and high endurance. The material- and device-level synapse innovations will be accompanied and integrated with innovations in circuit- and architecture-level techniques, toward an RRAM-based artificial intelligence computing platform.
“With vertical innovations across material, device, circuit and architecture,” said Seo, “we’ll pursue the tremendous potential and research needs toward energy-efficient artificial intelligence in resource-constrained hardware systems.”
The team envisions new materials- and device-based intelligent hardware that will enable a variety of applications with profound impacts.
“For instance, the intelligent information processing on power-efficient mobile platforms will boost new markets like smart drones and autonomous robots,” said Seo. “Furthermore, a self-learning chip that learns in near real-time and consumes very little power can be integrated into smart biomedical devices, truly personalizing health care.”
More Science and technology
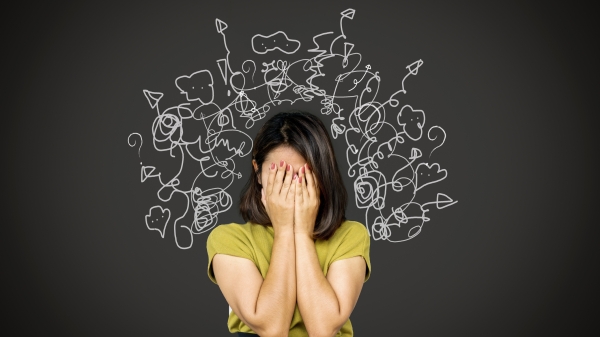
The science behind chronic stress
Stress comes in many shapes and sizes. There’s the everyday stress of preparing for a final exam or being stuck in traffic. And…
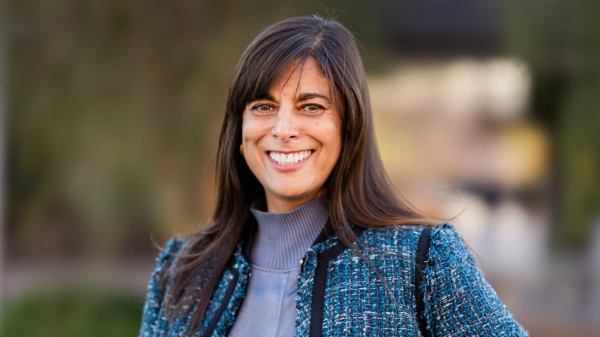
ASU planetary scientist to be inducted into the National Academy of Sciences
The National Academy of Sciences is inducting School of Earth and Space Exploration Director Meenakshi Wadhwa into the 2023 class…
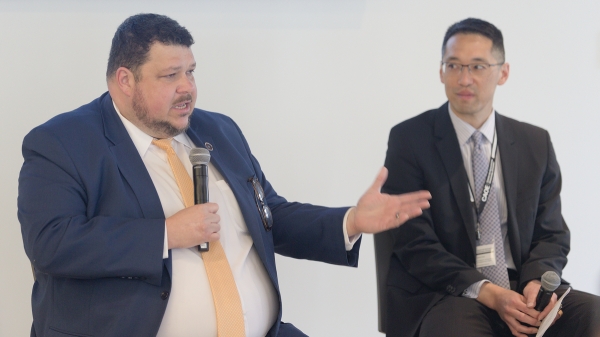
Unlocking the potential of AI for homeland security
“Can we do what we're doing now cheaper, more efficiently, more effectively?” Adam Cox, director in the Office of Strategy and…