ASU researchers develop tool to help determine a neighborhood's walkability
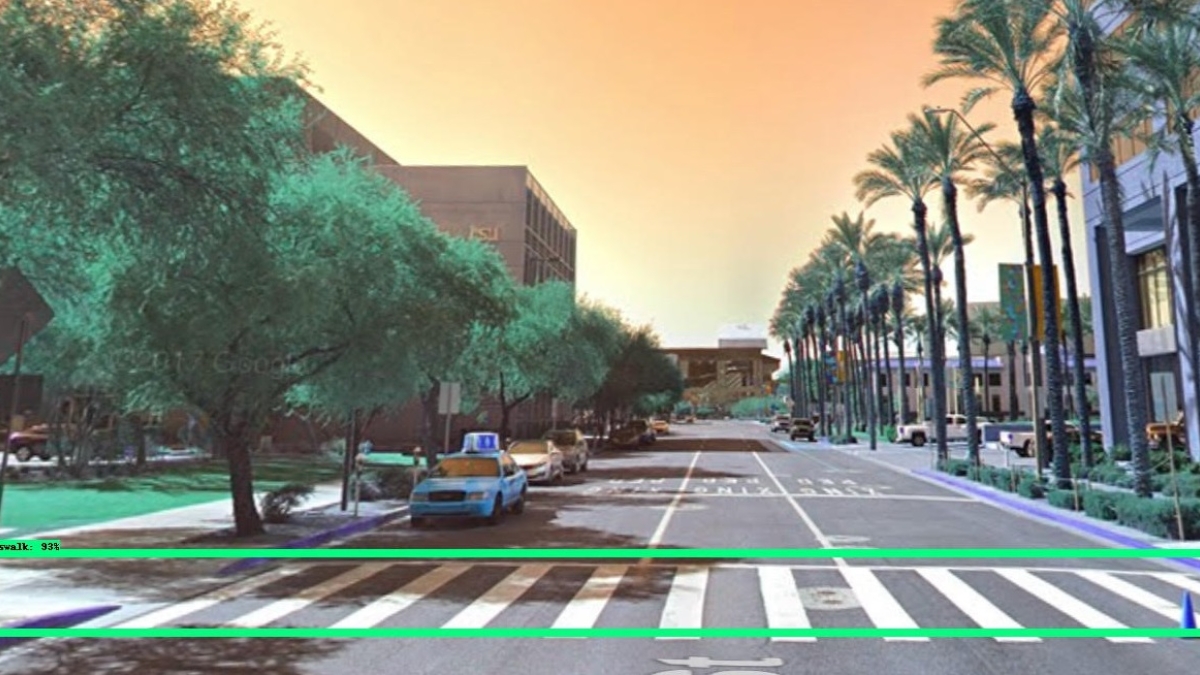
The new automated feature detection tool developed by ASU researchers is able to "see" neighborhood microfeatures like crosswalks, sidewalks, streetlights, trees and other characteristics, helping them more precisely determine a neighborhood's walkability.
You know you need to get more exercise. You want to be healthier. More physically fit. Mentally sharper. You know that regular physical activity can provide you with all these benefits, but so much gets in the way: lack of time, lack of motivation, your sedentary job, your neighborhood.
Wait. Your neighborhood?
Quite possibly. Behavior researchers have long known that a person’s surroundings have an effect on their physical activity levels, but exactly which neighborhood characteristics have the greatest impact is not clearly understood. Is it the large-scale neighborhood macrofeatures like street connectivity and residential density? Or do those smaller, microfeatures like sidewalks and bike lanes have the biggest influence?
The problem for scientists studying this issue is that it is difficult to effectively analyze built neighborhoods for both macro- and microfeatures. Large, publicly available geospatial data, the go-to, inexpensive tool they use to estimate macroscale walkability, doesn’t capture those microstructures, and sending people out to walk every neighborhood street to map all the sidewalks, streetlights and other minor features is expensive and time-consuming.
However, researchers at ASU’s College of Health Solutions, working with computer scientists at the School of Computing, Informatics and Decision Systems Engineering, are developing an automated, cost-effective tool that uses Google Street View (GSV), crowdsourcing, computer vision and deep learningDeep learning is a machine learning technique that teaches computers to do what comes naturally to humans: learn by example. In deep learning, a computer model learns to perform classification tasks directly from images, text or sound. to virtually detect a neighborhood’s microfeatures. They want to more precisely determine the correlation between the presence of certain features and the physical activity levels of its residents.
This automated microfeature detection system is part of a five-year study of 480 sedentary men and women in the Phoenix metropolitan area that is testing interventions that lead to increased aerobic activity. College of Health Solutions researchers Marc Adams, an associate professor, and Christy Phillips, a postdoctoral associate, are in the fourth year of “WalkIT Arizona.” The study outfits participants with wrist-worn activity monitors and also provides financial rewards, daily exercise goals and motivational text messages to encourage them. The next phase of their study will focus on the impact of their neighborhoods.
Adams and Phillips want to see how much the neighborhoods where study participants live affect how well they stick to their physical activity regimen.
“We enrolled participants over the last several years based on their various neighborhoods' macro level activity friendliness or walkability,” Adams said. “We are interested in seeing whether people who live in neighborhoods that are considered more walkable versus those who live in less walkable areas persist longer in their efforts after the year-long interventions end.”
This summer the National Cancer Institute at the National Institutes of Health awarded them close to $150,000 in supplemental grant monies to develop a way to detect microfeatures of the neighborhoods where their research participants live. They collaborated with Assistant Professor Ariane Middel, Associate Professor Ross Maciejewski and Akshar Patel, a graduate student, from the School of Computing, Informatics and Decision Systems Engineering to design and implement this automated neighborhood auditing tool. By combining GSV images with computer vision and deep learning techniques, their algorithms are “training” their computers to “see" microfeatures in online images, starting with their nearby surroundings, such as the crosswalk outside Adams’ and Phillips’ office building, which it detected with 99 percent accuracy (see photo above).
Being able to virtually map a neighborhood’s microfeatures on such a wide scale using a tool that is accurate, convenient and inexpensive will significantly increase understanding of how micro features affect regular, daily physical activity, Adams said.
“Evidence from previous studies suggests that microscale features are related to physical activity beyond what can be explained by macroscale walkability. However, we don’t know how these levels may interact with our behavior-change interventions. That’s why we think our work to develop an automated tool that is accessible and scalable could enable rapid advancement in this field.”
Adams and Phillips are excited about the far-reaching impact their study could have on infrastructure decisions made by city governments. For example, with more definitive data about the effect of minor features on physical activity, city planners might retroactively fit neighborhoods with sidewalks, pedestrian crossings, streetlights, crossing signals and trees to encourage physical activity because they are much cheaper than building connecting roads, parks and mixed-use developments.
“Macrofeatures are important to gauge an area’s walkability, but they probably don’t give us a full characterization of how walkable an area is and are very difficult to modify,” Phillips said. “Microscale features can be more easily and inexpensively modified to improve accessibility, safety, and the overall pedestrian and cyclist experience.”
They plan on sharing their open-source data, code and algorithms to more quickly advance the understanding of microscale features’ effect on neighborhood walkability which could inspire cities to enhance safety, increase physical activity and ultimately improve the health of its residents through neighborhood design.
“Maricopa Association of Governments has interactive online maps with various parks, bike lanes, trails and transit routes and stops, but as far as I know, they do not currently have a complete inventory of sidewalks throughout Maricopa County. We’d love to be able to give them the ability to do this at some point,” Phillips said.
While other research projects and applications have made extensive use of computer vision and online imagery, this study is unique in the way it has combined existing technology and a validated street audit instrument to create a new automated tool designed specifically to find features that directly impact physical activity. Adams and Phillips hope to have this phase of their study completed by October 2019 with results analyzed by 2021, when the WalkIT Arizona trial comes to an end.
More Science and technology
Extreme HGTV: Students to learn how to design habitats for living, working in space
Architecture students at Arizona State University already learn how to design spaces for many kinds of environments, and now they…
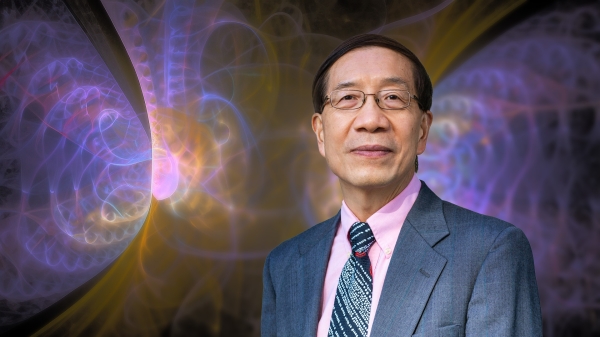
Human brains teach AI new skills
Artificial intelligence, or AI, is rapidly advancing, but it hasn’t yet outpaced human intelligence. Our brains’ capacity for…

Doctoral students cruise into roles as computer engineering innovators
Raha Moraffah is grateful for her experiences as a doctoral student in the School of Computing and Augmented Intelligence, part…