ASU student creates machine-learning model to identify neighborhoods most at risk for COVID-19
New research combines mobility patterns, social distancing data and social vulnerability data
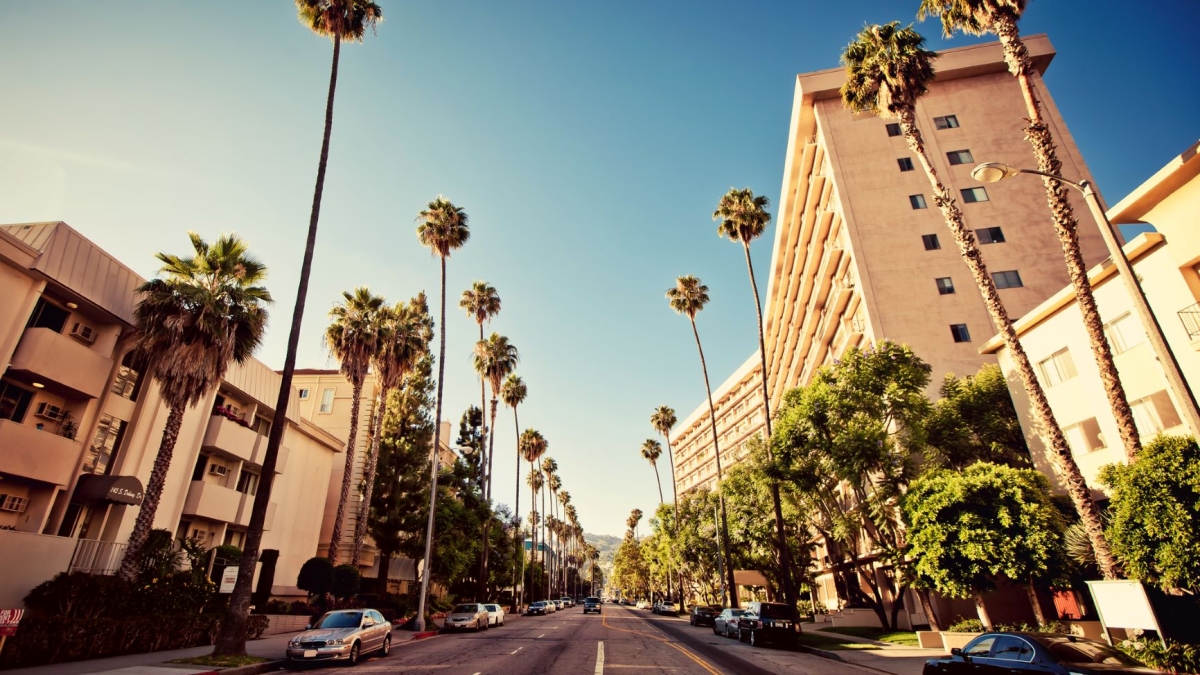
New research identifies the most at-risk populations down to the census block group level in Los Angeles. Photo courtesy of depositphotos.com
While health experts and government agencies across the globe continue to learn more about COVID-19 transmission and implement policies to curb its spread, a critical part of managing the disease is understanding with granularity which populations are at most risk and where these most vulnerable live.
New research led by Avipsa Roy, a graduate student in Arizona State University's School of Geographical Sciences and Urban Planning, quantifies this phenomenon using machine learning to identify the most at-risk populations down to the census block group level in Los Angeles.
In her paper titled “Characterizing the Spread of COVID-19 from Human Mobility Patterns and SocioDemographic Indicators,” co-authored by Bandana Kar from Oak Ridge National Laboratory, Roy leveraged anonymized human movement data from mobile phones and combined it with social distancing data and social vulnerability indicators to examine the overall spread of COVID-19 at local spatial scales.
Roy and Kar built a new machine learning model that analyzed all the data and predicted which census block groups would have the highest risk of COVID-19 cases based on new patterns teased out from their analysis.
“If people have less access to cars or health care, or if they are unable to visit hospitals to get tested, or if their neighborhood is in bad conditions, these are vulnerabilities that underlie certain population groups,” said Roy, who completed this research as part of an internship appointment with the National Science Foundation Mathematical Sciences Graduate Internship (NSF-MSGI) program. “We approximated these kinds of vulnerabilities through mobility and social vulnerability indices, and it helped our model predict the risk factors around COVID.”
“We predict whether the risk in each census block group is high, medium or low based on sociodemographic indicators along with mobility patterns of its residents before, during and after lockdown in LA.”
Roy says that understanding the COVID-19 pandemic at local scales is important for authorities to perform targeted testing and prevent and control the future spread of COVID-19.
“We developed a census block group level map indicator for local authorities to see and highlight which are the areas that need attention,” Roy said. “When COVID-19 first spread, no one knew where to test, how to test, or how much to test. This (research) can be used as an example map for authorities to plan ahead. When the third or fourth wave comes, what happens? Where do we go first?”
According to Roy, there are broader impacts of this model and it could be tailored for cities like Phoenix and beyond.
“We would like to extend this study to multiple cities especially those which are a global hot spot for informing local authorities on mitigation strategies for any future spread,” Roy said.
More Health and medicine
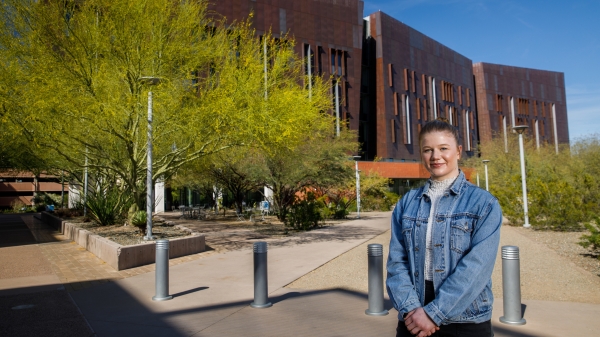
First exchange student for Biodesign Institute Europe bridges labs 5,000 miles apart
This spring semester, Grace Colley traveled to Arizona State University and became the first student to participate in the…
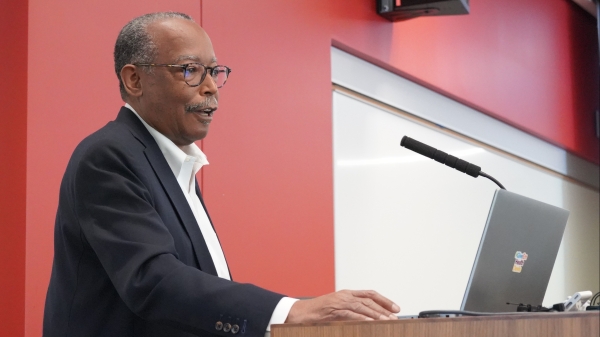
College of Health Solutions hosts visit from leading expert in genomic research
Some fortunate Arizona State University faculty, staff and students were able to gain valuable insights and perspective during a…
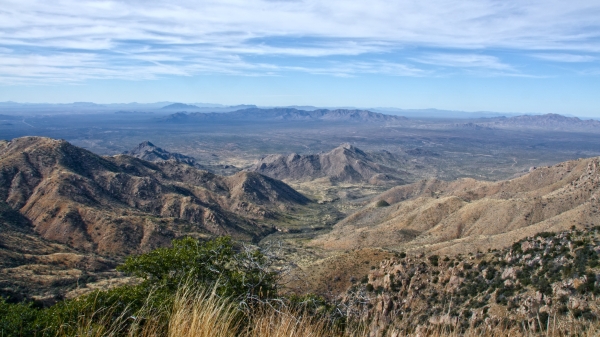
Indigenous ASU research team recommends assistance for tribal members still reeling from COVID-19’s effects
When Matt Ignacio’s tribe, the Tohono O’odham Nation, donated $1 million to Arizona State University to support COVID-19 research…