ASU research supports using AI for building healthy, safe transportation in cities

In today’s world of big data, billions of data points are collected every day via smartphone apps, fitness trackers and wearables about our decisions, habits and travel patterns. Already, this data has been the backbone for powering everything from self-driving cars to mobile banking to suggesting online retail advertisements.
But now, urban planning and geography researchers are seeking new ways to leverage this treasure trove of data to help inform transportation policy and infrastructure decisions that can benefit community health.
New research published in Transport Findings, led by Arizona State University PhD geography student Avipsa Roy and co-authored by Trisalyn Nelson, Foundation Professor in the School of Geographical Sciences and Urban Planning, and others, sought to develop a way to identify what kind of mode of transportation someone is using, solely based upon raw mobile GPS data and wearable accelerometer data, when combined with a specialized machine learning algorithm.
“The idea behind AI (artificial intelligence) is that it allows us to automate processes for large-scale planning operations with very little human intervention by accounting for measurement inaccuracies as well as gaps in data collection,” said Roy, whose field of research focuses on the intersection of computer science and urban planning. “Our research will enable planners to harness the power of big data for making strategic planning decisions by learning from existing data and thereby improving the safety of residents living in the city.”
Intersecting GIScience and urban planning
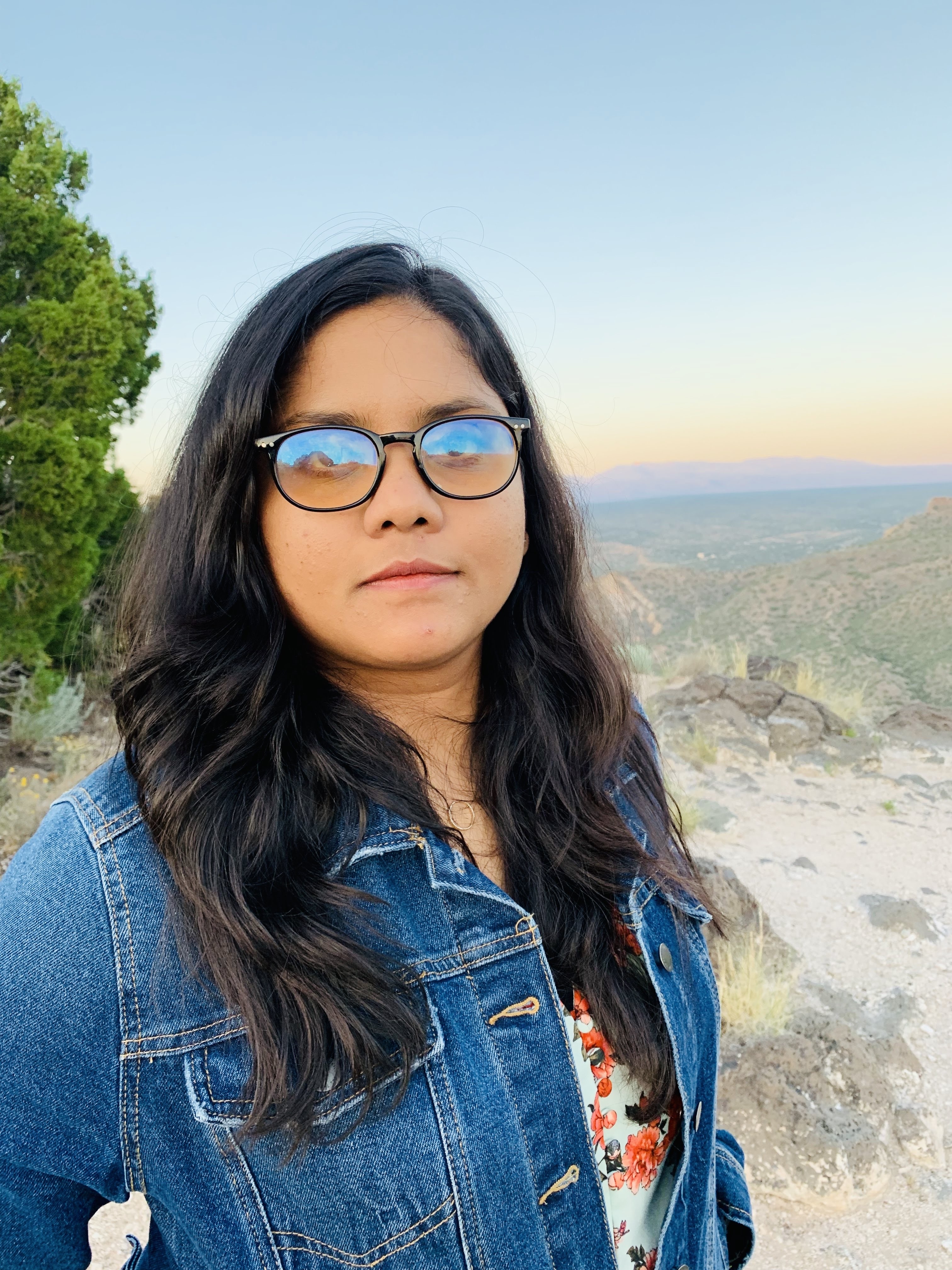
Avipsa Roy
In their research, Roy and her team analyzed raw phone GPS and wearable movement trajectory data captured by accelerometers from 12 anonymized users for a period of six months across two Canadian cities.
When run through a specialized machine learning algorithm that was tailored by Roy and her team, each trip extracted from the raw data could be classified into one of five different transportation modes — bicycle, bus, motor vehicle (cars/trucks), sky train (trams/light rail) or walking.
“We found that our model was able to classify the modes with 91.1% accuracy when data from both GPS and accelerometers were combined at an optimal temporal window,” Roy said. “Our method can be used to fuse human mobility data from different data platforms and derive actionable insights to accurately classify transport modes for mid-size North American cities.”
Nelson, co-author of the study and expert in spatial analysis and geographic information science says this research demonstrates how big data can be mobilized for urban planning.
“This work is helping develop the technology to allow us to monitor changes in travel behavior with GPS,” Nelson said. “These technologies are critical for making the link between the built environment and human health … it perfectly intersects GIScience and urban planning.”
The future of big data for urban planning
Research like that done by Roy and Nelson has many applications that are critical for transportation planning and monitoring the impact of existing policy and infrastructure.
“Specifically for active transportation modes like bicycles, practitioners lack sufficient information and tools to make targeted decisions about ridership behavior because they don’t know which areas are hot spots; why more crashes are happening, how it could be improved,” Roy said. “The methods we have developed can help them understand geographically — which are the locations of high ridership, low ridership, or even which are the locations where people, instead of bicycles, take more buses, cars, etc. — you can quantify the transportation footprint of the city and which transportation modes people are using on a daily basis.”
Although there are general privacy concerns associated with leveraging mobility data taken from apps and wearables, Roy says that individual privacy can still be maintained while researchers glean helpful data.
“A lot of anonymization algorithms are being introduced by data curators to obfuscate true locations and identities of people and their trajectories,” Roy said. “This will eventually cause less harm to individuals by preserving their location privacy and also help practitioners and researchers develop models for infrastructure management and reducing exposure to accidents.”
Top image courtesy of DepositPhotos.com.
More Science and technology

Lucy's lasting legacy: Donald Johanson reflects on the discovery of a lifetime
Fifty years ago, in the dusty hills of Hadar, Ethiopia, a young paleoanthropologist, Donald Johanson, discovered what would become one of the most famous fossil skeletons of our lifetime — the 3.2…

ASU and Deca Technologies selected to lead $100M SHIELD USA project to strengthen U.S. semiconductor packaging capabilities
The National Institute of Standards and Technology — part of the U.S. Department of Commerce — announced today that it plans to award as much as $100 million to Arizona State University and Deca…
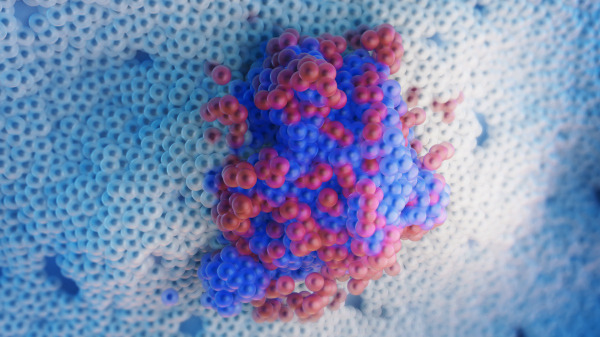
From food crops to cancer clinics: Lessons in extermination resistance
Just as crop-devouring insects evolve to resist pesticides, cancer cells can increase their lethality by developing resistance to treatment. In fact, most deaths from cancer are caused by the…